Successful plasma collapse avoidance experiment using machine learning
A control system to avoid plasma collapse phenomena in the Large Helical Device (LHD) has been developed using machine learning. Using this system, we have succeeded in avoiding radiative collapse in LHD experiments and maintaining a high-density plasma.
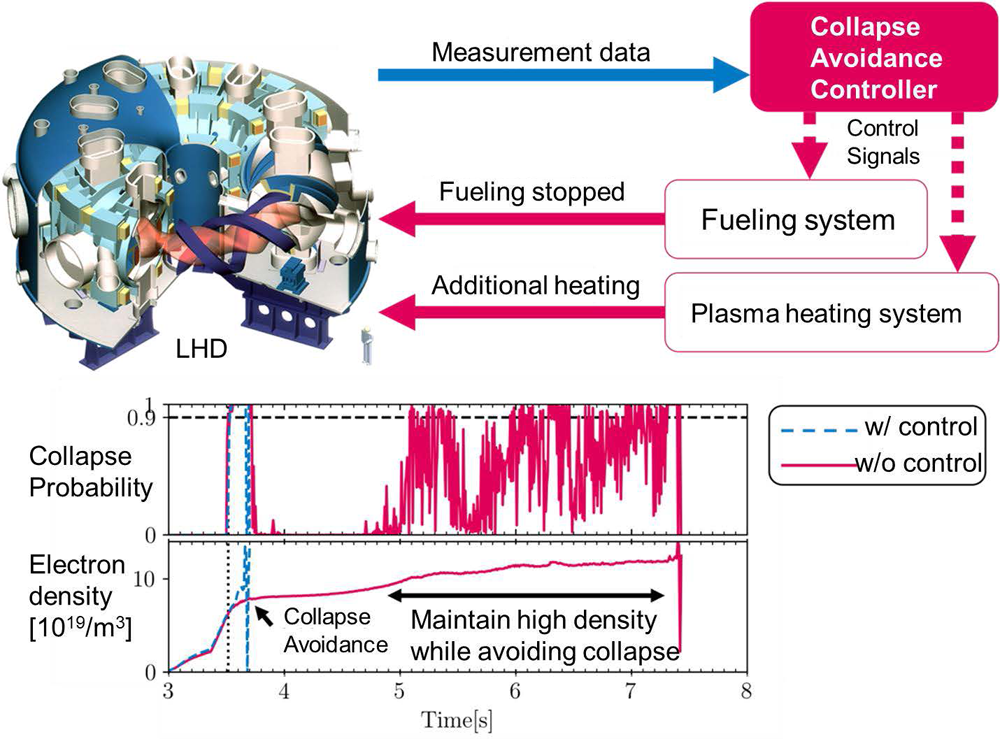
To realize fusion power generation, increasing the plasma temperature and density is necessary. However, the plasma suddenly disappears when the plasma density is increased, a phenomenon known as radiative collapse, which poses a problem. We report an experiment in which a machine-learning model for predicting the occurrence of the radiative collapse was incorporated into the plasma control system of the Large Helical Device (LHD) to control the fuel supply and heating of the plasma in order to avoid collapse.
A predictive model for the onset of radiative collapse was created using a support vector machine, which is a type of machine learning. The model was trained using data from LHD plasma experiments so that it could classify whether the plasma was in a stable or near-collapse state. The machine learning model was extended to a "probability of collapse" that is 0 when the plasma was stable and close to 1 as the plasma approached collapse. More details can be found in our previous work (T. Yokoyama, PFR, 2021).
The collapse avoidance control system is built around a small computer that serves as the controller. The controller receives measurement data directly from the LHD plasma, such as electron temperature, density, and impurity line radiation intensity, and calculates the probability of collapse in real-time. If the probability of collapse increases and the controller determines that the plasma is approaching collapse, it suspends the fuel supply to the plasma and simultaneously sends a signal to further heat the plasma. If the probability of collapse decreases and the plasma is judged to have avoided collapse, the signal is stopped and the plasma returns to normal discharge.
We applied this collapse avoidance control system to an actual LHD discharge experiment to investigate its performance. In the experiment, the system successfully avoided a collapse that was about to occur in the early stages of the discharge. The density was maintained as high as 1.2×1020 m-3 by applying fine control each time a collapse approached, and we were able to show the first example of avoiding radiative collapse in a helical plasma such as that in the LHD by using machine learning control.
This work was supported by Grant-in-Aid for Challenging Exploratory Research (19H05498) and Grant-in-Aid for Young Scientists (19J20641) for "Study of sudden collapse phenomena in fusion plasmas by a data-driven approach". The research group of Suguru Masuzaki and Ryuichi Sakamoto at the National Institute for Fusion Science, in collaboration with Hiroshi Yamada and Tatsuya Yokoyama at the Graduate School of Frontier Sciences, The University of Tokyo (currently, National Institute of Quantum Science and Technology).
The results of this research were published in Plasma and Fusion Research, an online journal of the Plasma and Fusion Society of Japan, on March 30, 2022.