Predicting the onset of plasma collapse and capturing the changes in the plasma as it moves toward collapse
Using experimental data from the Large Helical Device (LHD), we evaluated the probability of radiative collapse using machine learning techniques. We also clarified how plasma changes during a time when the probability of collapse increases, i.e., when the plasma is approaching collapse, through simulations.
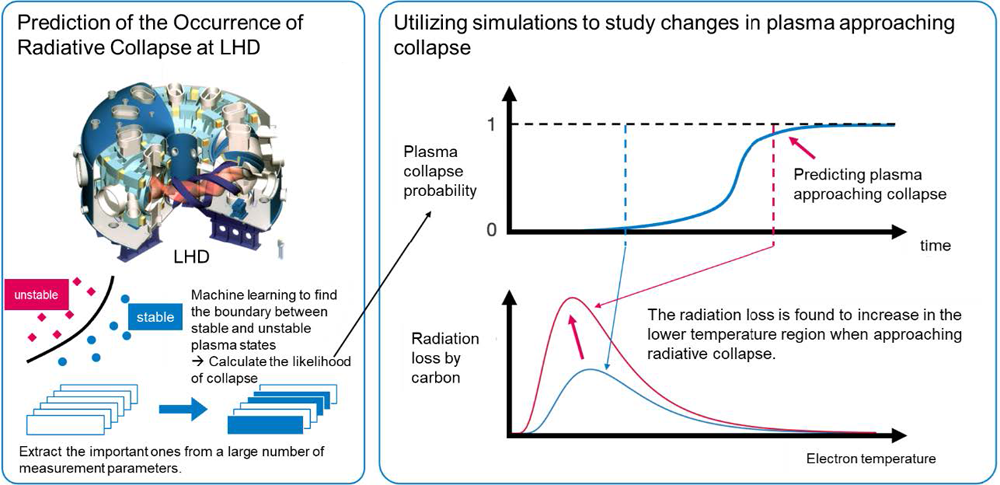
To realize fusion power generation, increasing the temperature and density of plasma is necessary. However, the plasma suddenly disappears when its density is increased, a phenomenon known as radiative collapse. In this study, we have developed a model that predicts the occurrence of radiative collapse using a data-driven method, which enables us to calculate the probability of the occurrence of collapse. Furthermore, by simulating the plasma during the time when the probability of collapse increases, we have clarified the changes that occur as the plasma moves toward collapse.
In this research, "support vector machines," a type of machine learning, were trained on experimental data from the Large Helical Device (LHD) to classify whether the plasma was in a stable or near-collapse state. To collect data from a wide range of conditions to broaden the range of applicability of the predictor, experiments were conducted under 2 × 2 = 4 different conditions: hydrogen or deuterium plasma, and a strong or weak confinement magnetic field. The machine learning classification was extended to the "probability of collapse", which is 0 when the plasma is stable and takes a value close to 1 as it approaches collapse, to enable the evaluation of how close the plasma is to collapse.
The combination of measurement parameters used for classification has been optimized by introducing sparse modeling, a data-driven method. The line-averaged electron density, the electron temperature at the plasma edge, and the line emission intensity of carbon and oxygen impurities were extracted as parameters that characterized the onset of radiative collapse, suggesting that the behavior of impurities in the relatively low temperature (tens of eV) region at the plasma edge was the key to the onset of the collapse.
To investigate the behavior of impurities in the surrounding region, simulations were performed using the EMC3-EIRENE code, which calculated the transport of plasma and neutral particles in three-dimensional space. The results were compared before and after the "probability of collapse" increased, and it was found that the plasma energy loss due to carbon impurities, corresponding to the features extracted from the sparse modeling, increased, especially in the lower temperature region. Thus, we were able to capture the plasma changes leading to radiative collapse through "data-driven science," whose methods are utilized in discussions of plasma physics.
This work was supported by Grant-in-Aid for Challenging Exploratory Research (19H05498) and Grant-in-Aid for Young Scientists (19J20641) for "Study of sudden disruption phenomena in fusion plasmas by the data-driven approach". The research group of Suguru Masuzaki and Ryuichi Sakamoto at the National Institute for Fusion Science, in collaboration with Hiroshi Yamada and Tatsuya Yokoyama at the Graduate School of Frontier Sciences, The University of Tokyo (currently, National Institute of Quantum Science and Technology).
The results of this research were published on February 26, 2021 in Plasma and Fusion Research, an online journal by the Japan Society of Plasma Science and Nuclear Fusion Research.